Wax Prediction in Oil and Gas Flow Assurance
Tackling Flow Issues, Equipment Failures, and Production Loss
Understanding Wax Precipitation Challenges
Wax precipitation poses significant flow assurance challenges in the oil and gas industry, leading to flow issues, equipment failures, and production loss. This complex process involves both phase separation and solidification, making accurate prediction crucial for well intervention.
ABOUT THE PRODUCT
Our solution leverages Perturbed Chain Statistical Associating Fluid Theory (PC-SAFT) to predict wax precipitation accurately, eliminating the need for costly PNA analysis. PC-SAFT excels in estimating the frugalities of heavy components in vapor and liquid mixtures. To address variations in wax composition across the field, we divide the reservoir into sectors with similar wax properties, calibrating each sector separately for precise predictions.
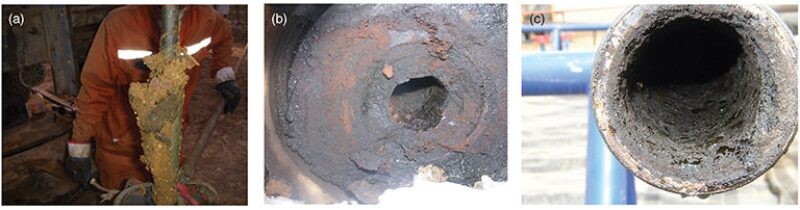
KEY BENEFITS
• Accurate Predictions: Ensure precise prediction of wax precipitation for each well, preventing flow issues and production disruptions.
• Sector-specific Calibration: Customize predictions by calibrating PC-SAFT parameters for each reservoir sector, enhancing accuracy.
• Risk Identification: Utilize a Feed-Forward Neural Network with Distributed Temperature Sensing (DTS) data to identify high-risk wells, enabling proactive intervention.
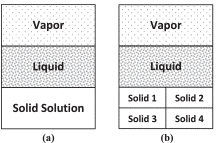
METHODOLOGY
Our methodology combines PC-SAFT, sector-specific calibration, and a Feed-Forward Neural Network trained on DTS data. The heat transfer equation is applied to model radial temperature gradients, enhancing the accuracy of the PC-SAFT model. Sensitivity analysis identifies critical parameters affecting wax precipitation, emphasizing temperature as a dominant factor.
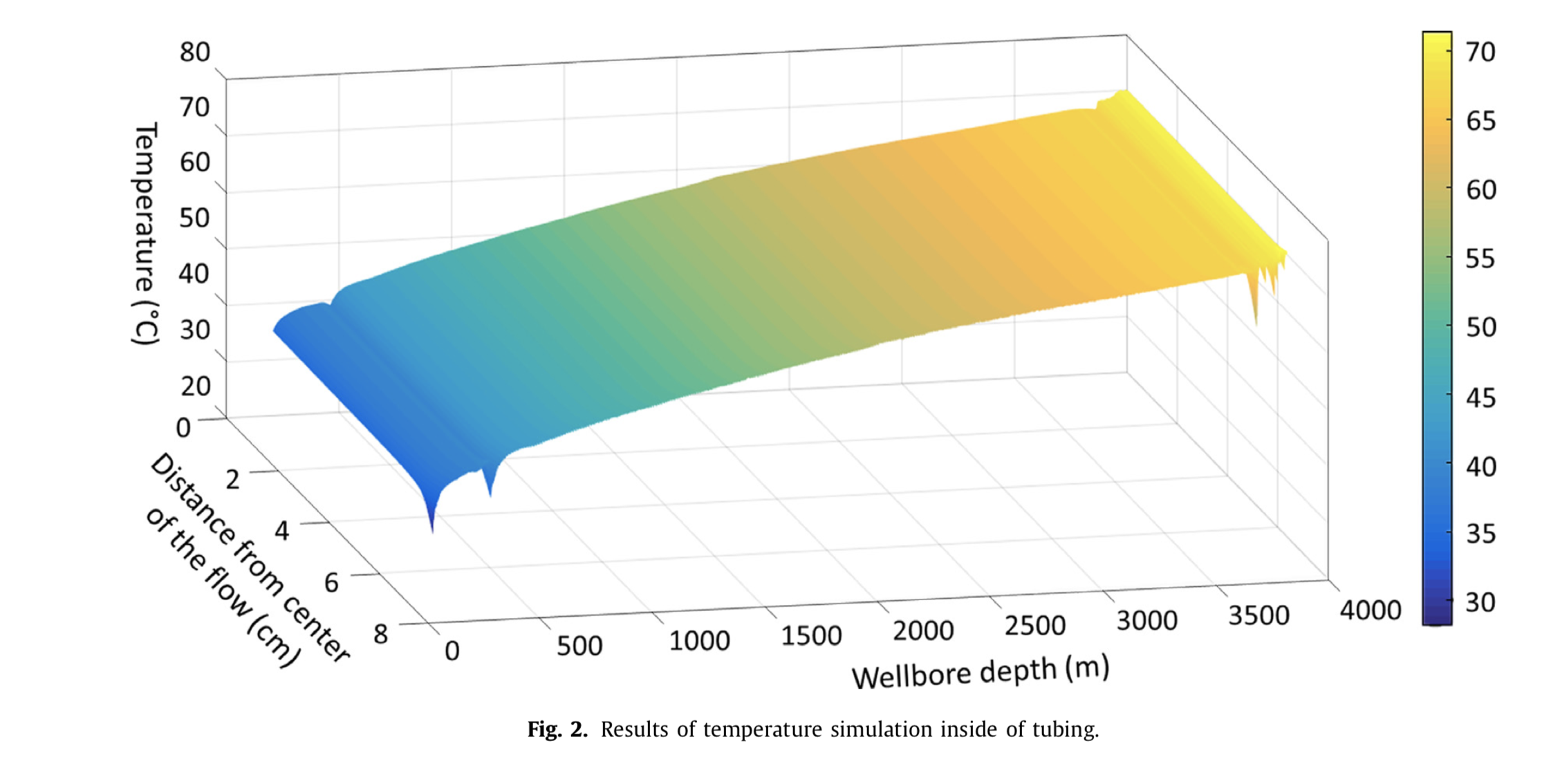
Our product advances the understanding of solution-solid and multi-solid models in conjunction with the PC-SAFT Equation of State, integrated into a three-phase flash calculation algorithm. The developed thermodynamic model employs sensitivity analysis to highlight key parameters like gas/oil ratio (GOR), pressure, and, notably, temperature, with potential wax precipitation increases of 2–4 times depending on temperature drops. This comprehensive approach allows engineers to proactively address wax precipitation risks before well intervention.