Pre-Stack Seismic inversion based on Continuous Wavelet Transform Processing and Deep Neural Network
Seismic inversion is one of the critical issues for geophysicists in the oil and gas industry. It is commonly used to estimate the distribution of facies-types and fluids in reservoirs. The importance of this operation is that it is actively used in a chain from exploration to development to reduce drilling uncertainty and enhance hydrocarbon recoveries. Also, it is valuable for well placement and production prediction. However, a large number of uncertainties make direct use of inversion results challenging.
Our research team used the Continuous Wavelet Transform to predict the 3D volume of Gamma Ray data from pre-stack seismic reflectivity partial angle stacks using the Convolutional Neural Network architecture.
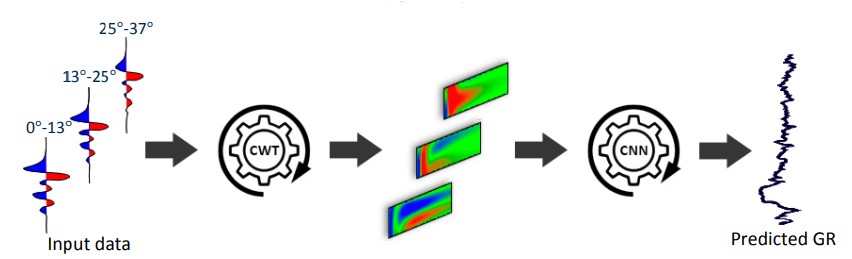
132 wells from the Azeri and Chirag sectors of the ACG field in the South Caspian Basin were used as input to the algorithm as training data.
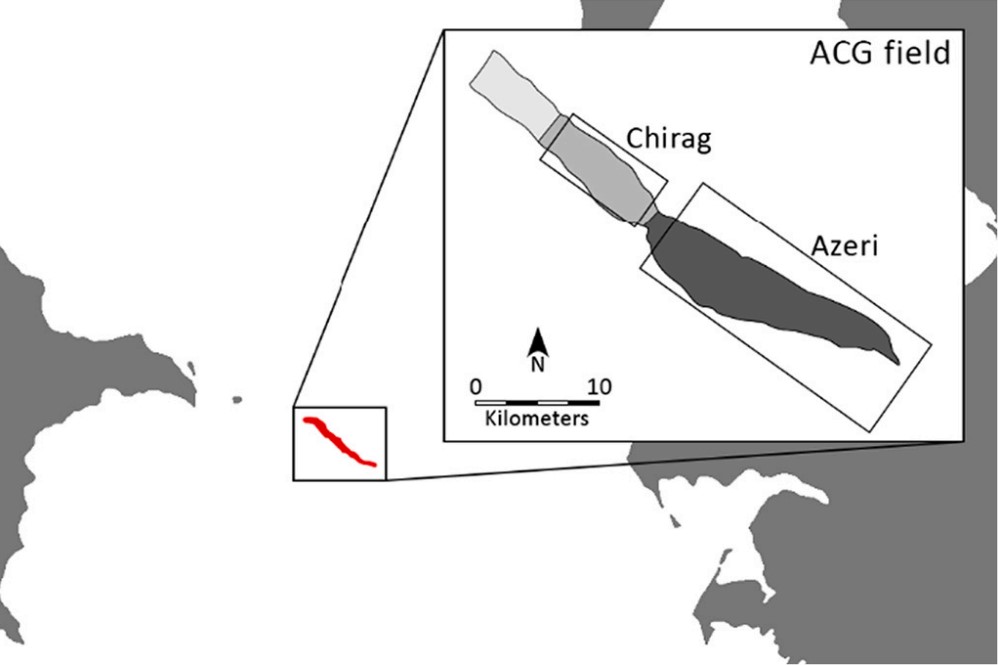
The database included pre-stack seismic reflectivity data from the field at a limited vertical resolution ca. 20 meters and 132 wells with various levels of deviation.
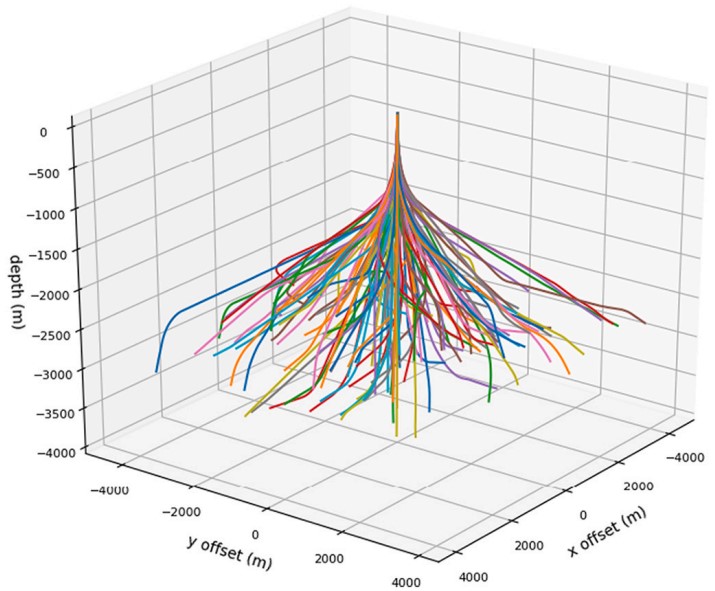
To deal with high well deviation, the concept of a representative seismic trace (RST) was proposed. The RST is a trace that passes through the location of the log point and is created by a weighted average of seismic traces that are located in a circle with a radius of 6.25 m and centered in the log point coordinates.
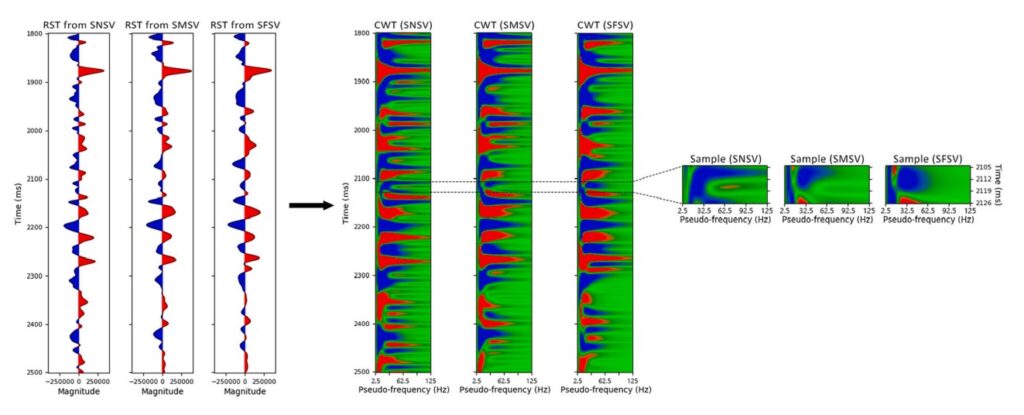
final cropped samples of SNSV, SMSV and SFSV for one log point (three right graphs)
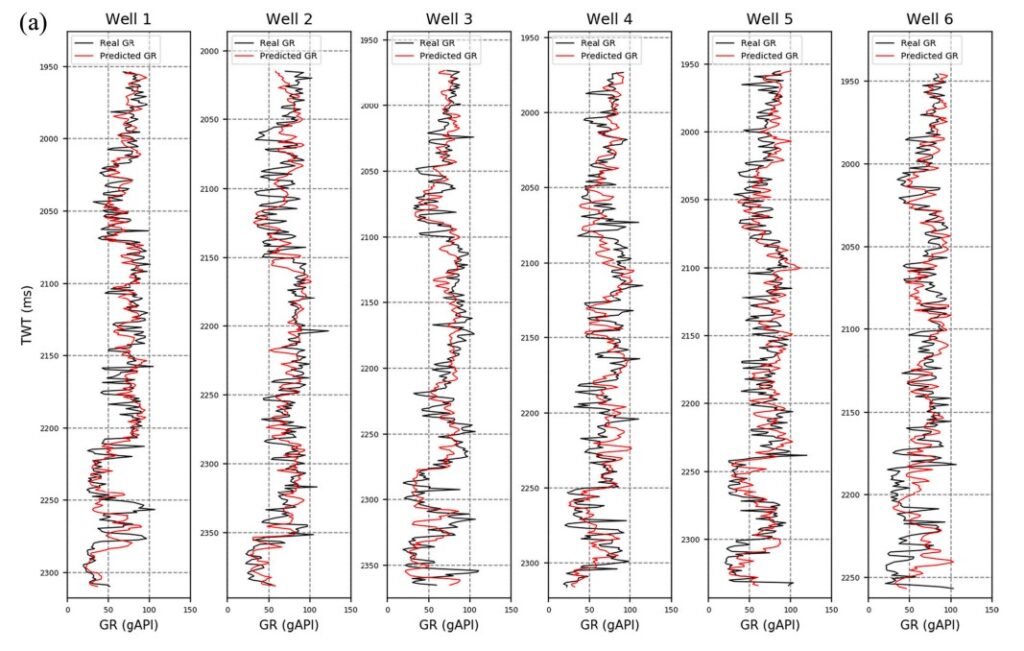
The black line and red line show the real and predicted GR log, respectively
One of the methodology’s benchmarks in testing blind wells revealed promising Gamma Ray predictions. The proposed methodology exhibited the capacity to accurately predict Gamma Ray across the fields, and eventually the methodology was deployed on the whole project field to predict the 3D GR cube. The results have the potential for use in well placement optimization and reservoir modeling. We intend to test this methodology separately for prediction of other physical rock properties (bulk density, true resistivity, compressional velocity, etc.) or by feeding the network with several well logging data types in addition to seismic to more accurately tie the seismic with the aforementioned properties..
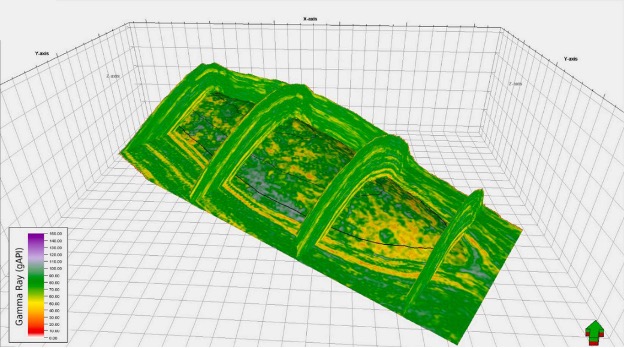